How to accelerate your AI/ML initiatives by integrating with a knowledge-driven approach

New technologies based on Artificial Intelligence are now making significant inroads into the Energy Sector, especially within the real-time, data-heavy domains of operational drilling and reservoir surveillance. AI/ML algorithms are also playing an ever more prominent role in the predictive maintenance of facilities, in reservoir modelling and production forecasting.
With access to modern web-based tools and continual increases in processing speed, combined with the versatility of cloud computing, the oil industry’s adoption of Artificial Intelligence looks set to vastly improve existing efficiencies.
During a recent project, Flare Solutions outlined one of the key differences between data driven and knowledge driven approaches to developing AI/ML algorithms.
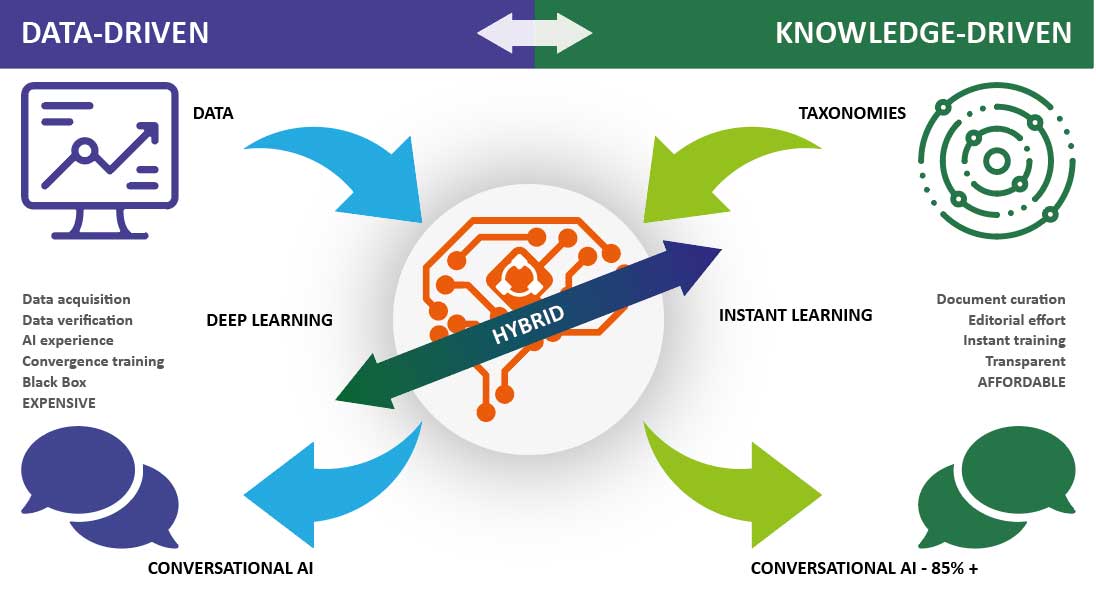
An international energy company was conducting its own research and development into AI/ML tools to enhance data extraction and verification. However, the tool-set required significant input for specific, narrow use-cases and was missing opportunities for data acquisition and verification due to a lack of consistent terminology within data sets.
Flare’s Taxonomies with their standard reference terms and synonyms, for keywords, asset names and deliverables can deliver a step-change in understanding the terminology. This means the AI system starts with a University level of industry knowledge rather than a primary school one. Leveraging this knowledge reduces the need for complex deep learning.